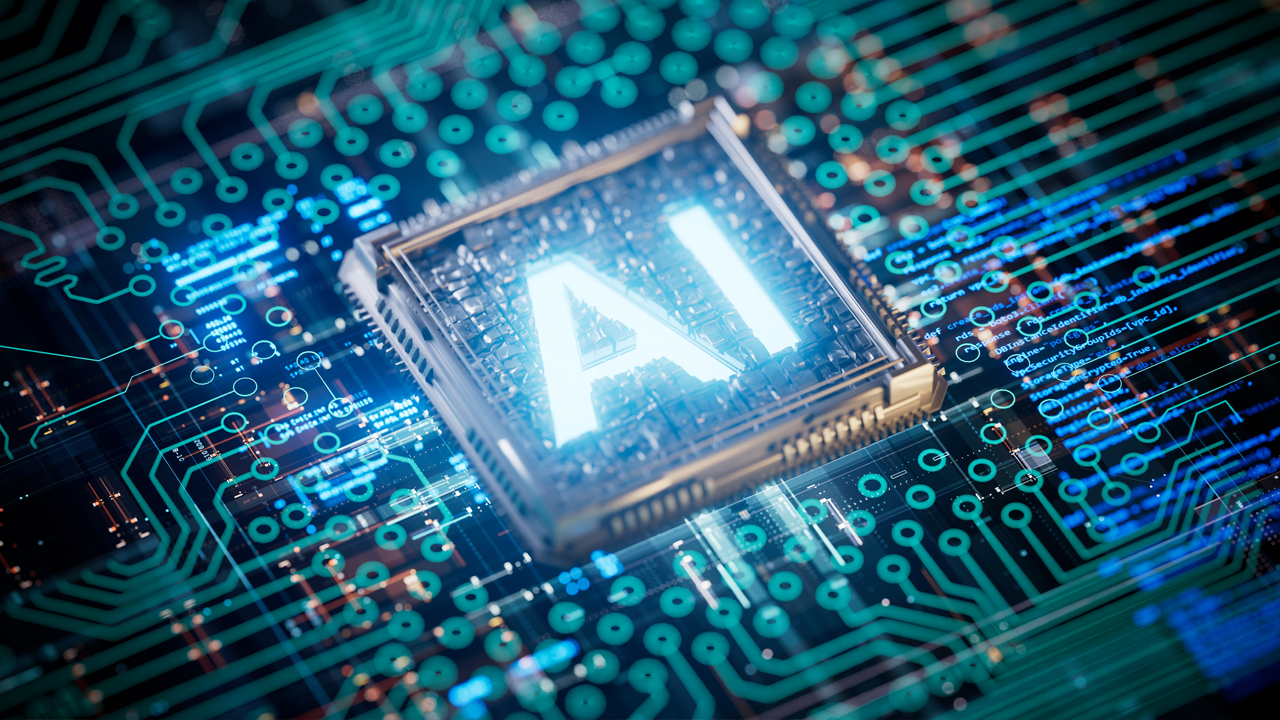
Q: How do AI-based models like GenCast compare to traditional weather forecasting methods in terms of speed and accuracy, and what potential do you see for their integration into meteorological practices?
A: Let me begin with a quick note on terminology. Most modelers prefer to describe models like GenCast, which is mentioned in this story, as machine learning-based (ML-based) rather than AI-based. ML, a subset of AI, enables the creation of highly accurate statistical models by training numerous parameters on large datasets. I suspect that Google uses the broader term because it may work better for publicity.
As for speed, ML-based models like GenCast tend to be faster than a traditional physics-based model. The main advantage, however, is in the cost rather than the speed.
Training an ML-based forecast model involves teaching it to make predictions by analyzing large datasets, which requires high-performance computing (HPC) resources. However, this training process is only done once. Once the ML model is trained, it can be used repeatedly to generate forecasts without requiring HPC resources again. In contrast, traditional weather prediction models rely on HPC systems for each forecast, running new simulations in real time. This ongoing dependence makes traditional models much more resource-intensive in terms of cost and energy. ML-based models, once trained, can operate efficiently on less powerful and more affordable machines, making them a more sustainable option for weather forecasting.
Keep in mind, however, that there is a substantial hidden cost component: the high-quality ML-based models rely on European Centre for Medium-Range Weather Forecasts (ECMWF) data for training and to start forecasts. This data has been produced with the help of traditional models that are the products of decades of research and development by the atmospheric science community. Note also that an operational center like ECMWF collects billions of daily real-time weather observations and uses tens of millions of them to prepare the data used to train and start the current ML-based models.
As for accuracy, I am not convinced that the current ML-based models can significantly outperform the traditional models since they heavily rely on data produced by conventional models. These ML-based models have much lower temporal resolutions than traditional models. The 12-hour resolution of GenCast is acceptable for a 15-day forecast, but it would not be for a one- to three-day, high-resolution forecast. Some AI-based models have a temporal resolution of three to six hours, but that is still a far cry from the temporal resolution of the traditional operational models. Current ML-based models also do not have demonstrated forecast skill beyond the 15 days range of GenCast, while the best traditional models can provide useful forecast information for months.
Q: Extreme weather events are becoming more frequent and intense due to climate change. How can advanced AI-driven systems improve early warnings and preparedness for such events, particularly in vulnerable regions?
A: Purely ML-based models are probably not the best tools for this. First, these models are trained on data representing the climate of the past 30 years. They may not work well in weather conditions associated with a changed future climate. Second, ML-based models still struggle with simulating the current climate. Most likely some combination of traditional and ML-based modeling will provide the best results, because the universal laws of physics that are the backbone of traditional modeling will always be valid independently of the changes of the climate.
Q: According to the AFP article, the ECMWF's forecasting model is widely regarded as the global standard. What challenges arise when we incorporate AI-driven models like GenCast into established forecasting systems, and how can those be addressed?
A: ECMWF has had the best forecast models in the world since it began operations on Aug. 1, 1979. The secret of its success is that it has always been a forward-looking organization that is actively looking for new ideas. Not surprisingly, it has been the leader among all the operational weather prediction centers in exploring the potential of ML-based models. It runs ML-based forecast models for big-tech companies in-house and makes forecast data from these models publicly available for comparison. It has also developed its ML-based model called the Artificial Intelligence Forecasting System (AIFS), which integrates advanced ML techniques to produce accurate and efficient weather predictions.
Q: In your opinion, what role will AI play in forecasting extreme events like hurricanes or wildfires, and what improvements are most urgently needed in these areas?
A: AI (and not just ML) has massive potential in these areas. A weather prediction model predicts weather parameters. Still, the interpretation of the predicted parameters for the public and even for many professional users is done by human experts (think of the experts on the Weather Channel in the case of a hurricane). While I am not saying that AI could completely replace human experts, it will enhance the predictions of the impact of weather on society. In addition, the prediction of wildfires relies on models that use forecast information from the weather prediction models as input. These models already benefit from the ML-based approach. ECMWF has published an insightful blog post exploring the impact of machine learning on wildfire prediction.
Q: What ethical or societal considerations should scientists and policymakers consider when developing and deploying AI-based weather forecasting systems, especially in climate change adaptation?
A: I do not see any obvious ethical issues as of right now. It is clear, however, that ML has the potential to become a disruptive technology for the weather prediction enterprise in the U.S. Until now, the collection of weather observations and the preparation of model forecasts has been primarily performed by government entities. The data these entities produced have been freely available for research and commercialization. Developing traditional weather prediction models was not attractive to for-profit companies because it required years of work by a large group of highly trained scientists (often done as a community effort by government and academic scientists) and burned a large amount of HPC computer time. Developing an ML-based model turned out to be much easier. It takes only a few domain scientists and a group of software engineers to quickly build a highly accurate model if high-quality data is available for model training and to start the forecasts. Since most software engineering problems are not unique to such models, there is a lot of flexibility regarding the workforce. It’s not surprising that building forecast models has become much more attractive for big-tech companies. Thus far, they have mainly used these models to publicize their AI capabilities or demonstrate the power of their hardware. In this case, NVIDIA, a leading tech company specializing in graphics processing units (GPUs), uses its hardware to accelerate the ML-based forecast models, showcasing its ability to handle complex computational tasks like weather prediction.